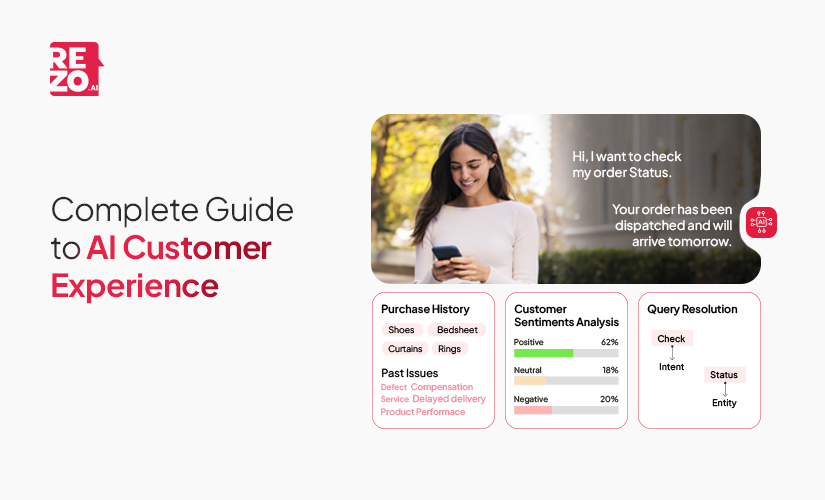
Complete Guide to AI Customer Experience
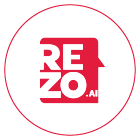
Complete Guide to AI Customer Experience
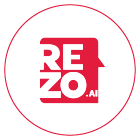

Artificial Intelligence (AI) is now a key component in modern customer experience (CX) strategies. For customer experience leaders and C-suite executives, AI means tangible solutions to meet rising customer expectations while reducing operational costs.
AI customer experience includes machine learning algorithms, natural language processing and predictive analytics that enhance customer interactions across all touchpoints. These technologies allow you to personalise experiences, automate customer queries and gain insights from customer data.
Gartner says that by 2025, 80% of customer service and support organizations will be applying generative AI technology in some form to improve agent productivity and customer experience. (Source)
As digital interactions continue to own the customer journey, AI gives you the tools to scale personalisation without the cost.
This guide provides a practical framework for understanding, implementing and optimising artificial intelligence in your customer experience strategy. It covers key considerations for technology selection, implementation approaches and measurement methodologies to ensure your AI investments deliver business outcomes.
Understanding the Role of AI in Customer Experience
AI serves several purposes in the customer experience ecosystem, each addressing different parts of customer engagement and operational efficiency.
Machine learning algorithms analyse customer behaviour to understand preferences and predict future needs. These get better with time as more data is processed. Natural Language Processing (NLP) powers conversational interfaces that understand customer queries and respond accordingly whether through chatbots, voicebots, virtual assistants or voice recognition systems.
Predictive analytics applications forecast customer behaviour, identify churn risks and recommend next best actions for service agents or even sales teams. Computer vision technology enhances visual search and automated quality control in product experiences. Robotic Process Automation (RPA) automates back office operations that impact customer experience like order processing and account management.
The combination of these technologies creates an AI powered CX framework that operates across three dimensions:
- Customer understanding: AI systems gather data from multiple sources to create customer profiles beyond traditional segmentation.
- Operational intelligence: AI allocates resources, identifies process inefficiencies and automates routine tasks to improve response times.
- Experience delivery: AI enables real-time personalisation across channels, consistent information delivery and proactive service interventions.
This is a big departure from traditional CX approaches. While traditional systems rely on historical reporting and manual interventions, AI powered customer experience runs continuously, adapting to changing customer behaviour and business conditions without needing to be reconfigured all the time.
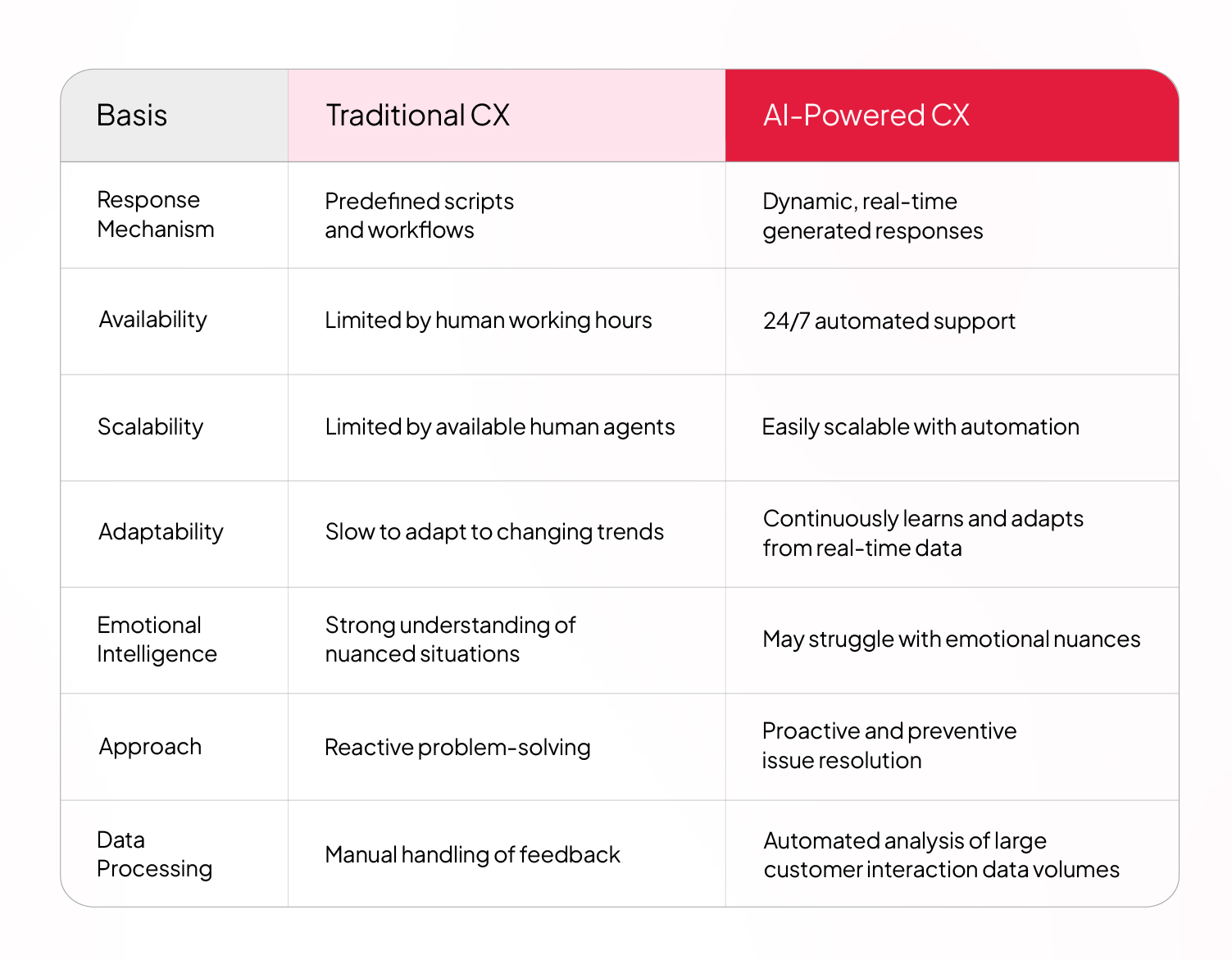
Key Benefits of AI in Customer Experience
AI delivers measurable advantages for customer experience strategies across multiple dimensions. These benefits directly impact operational efficiency, customer satisfaction, and revenue generation.
Personalisation at Scale
AI turns standard customer interactions into highly individualised experiences by crunching vast amounts of customer data. Machine learning algorithms find patterns in browsing behaviour, purchase history and demographic info to create detailed customer profiles. These profiles allow businesses to serve up tailored content, product recommendations and service experiences across all touchpoints.
The impact of AI personalisation goes beyond just product recommendations. AI can adjust tone of voice and prioritise features based on individual preferences. This level of personalisation was previously impossible at scale, would require too many staff to do manually.

Organisations using AI personalisation can increase revenue through higher conversion rates and bigger average order values. Customer engagement metrics also go up with massive increases in time on site or app and return visit frequency. Most importantly personalisation addresses the needs of customers who abandon brands after having a generic one-size-fits-all experience.
Intelligent Virtual Agents
Chatbots and virtual assistants have gone from simple rule based systems to conversational interfaces that can resolve complex customer queries. They provide 24/7 support across multiple channels, handling routine questions and transactions without human intervention.
AI assistants use natural language processing to understand customer intent even when the question is poorly phrased or has multiple requests. They keep context across interactions and can hand off to human agents when needed, and pass all the relevant information so it’s continuous.
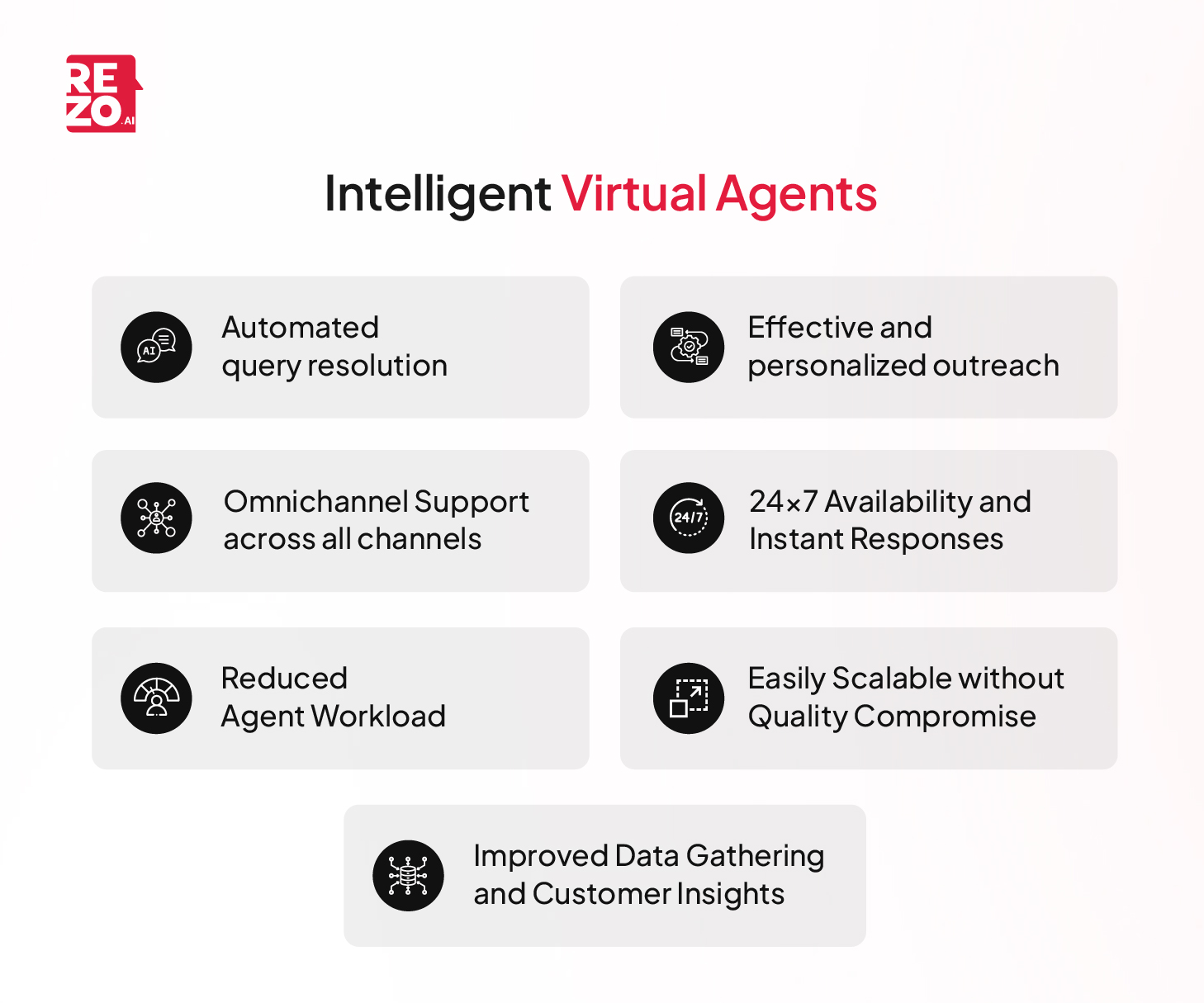
Self service options powered by AI can resolve up to 80% of common customer questions, reducing wait times from minutes to seconds. This is especially valuable during high volume times like product launches or seasonal peaks when staffing would otherwise be a bottleneck. Companies that implement self service with AI can reduce support costs while maintaining (or improving) customer satisfaction metrics.
Omnichannel Integration
AI aggregates customer data across channels, so customers have the same experience no matter how they interact with a business. This means seamless transitions between digital channels (website, mobile app, social media) and traditional channels (phone, in-store) and conversation context.
AI tracks customer journeys across touchpoints, so information provided in one channel is available in others. No more repeating information when switching channels and continuous conversations across multiple sessions.
Sentiment Analysis and Emotional Intelligence
AI customer experience platforms can analyze customer communications across text, voice and social media to detect emotional states and satisfaction levels. Natural language processing detects subtle cues in word choice, sentence structure and tone to go beyond explicit statements.
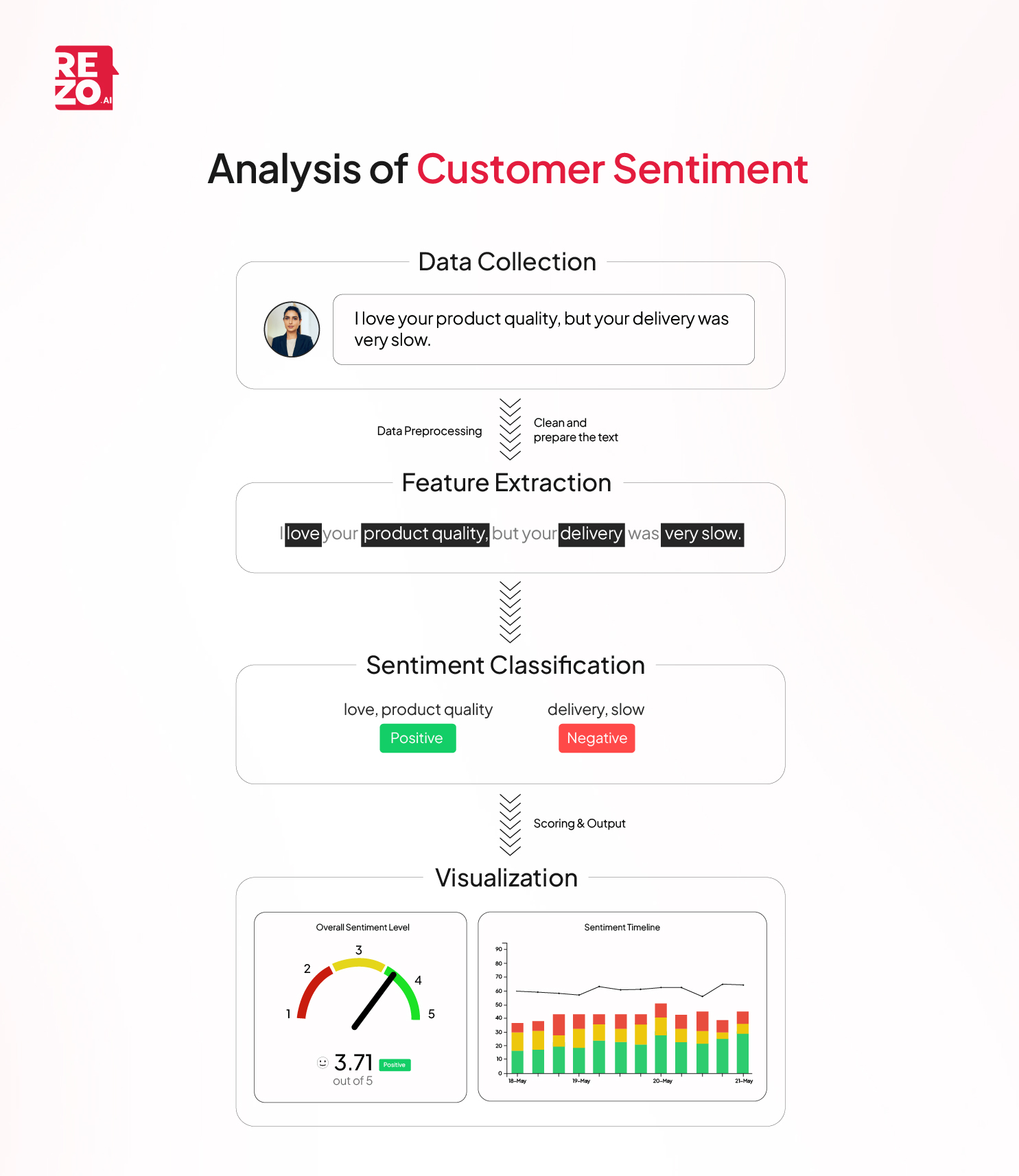
This allows for proactive intervention for at-risk customers before formal complaints happen. Support teams can prioritize responses to negative sentiment interactions and adjust their approach based on the customer’s current state.
Sentiment analysis also detects emerging issues and trends that may not show up in structured feedback, so organizations can address systemic problems before they impact broader customer segments.
Advanced AI customer experience systems now recognize emotional nuances beyond simple positive/negative classifications, detecting frustration, confusion, satisfaction or delight. This kind of intelligence allows for more appropriate responses and can trigger specific workflows based on detected sentiment.
Real-Time Experience Modification
AI adjusts digital experiences in real-time based on customer behavior and context. Content, offers and navigation paths adapt to individual preferences and needs during active sessions, creating dynamic experiences that respond to changing customer intent.
For example, AI can detect hesitation during checkout and offer help or incentives to complete the purchase. Real-time adjustments also support accessibility needs and device-specific optimizations.
Being able to identify struggling users in real-time allows for intervention before abandonment happens. AI recognizes patterns of confusion or frustration and businesses can provide timely support to resolve issues while the customer is still engaged.
Enhanced CRM Capabilities
AI customer experience platforms add to customer relationship management systems by automating data entry, finding sales opportunities and prioritising follow up actions.
AI powered CRM systems analyse customer interactions to find patterns and relationships that human analysis might miss. They can predict which customers are most likely to convert, which products they’ll be interested in and when to reach out.
The efficiency gains mean more meaningful customer interactions and higher sales productivity.
Data-Driven Decision Making
AI customer experience analytics turn vast amounts of customer data into actionable insight for strategy. They find correlations and causal relationships that might be hidden in traditional analysis and provide clear recommendations based on full data analysis.
Decision makers get AI generated insights that combine quantitative metrics with qualitative factors like sentiment and behaviour. This gives a more accurate forecast and strategy than traditional reporting.
AI isn’t just about automating tasks, it’s changing how businesses interact with data. Instead of pulling endless reports or manually analysing user sessions, AI can find key trends, summarise insights across multiple sessions and pinpoint the most important opportunities – all in real time.
Challenges of Implementing AI in Customer Experience
Implementing AI in customer experience offers significant benefits, but organizations must navigate several challenges to realize its full potential. Understanding these obstacles is essential for developing effective implementation strategies.
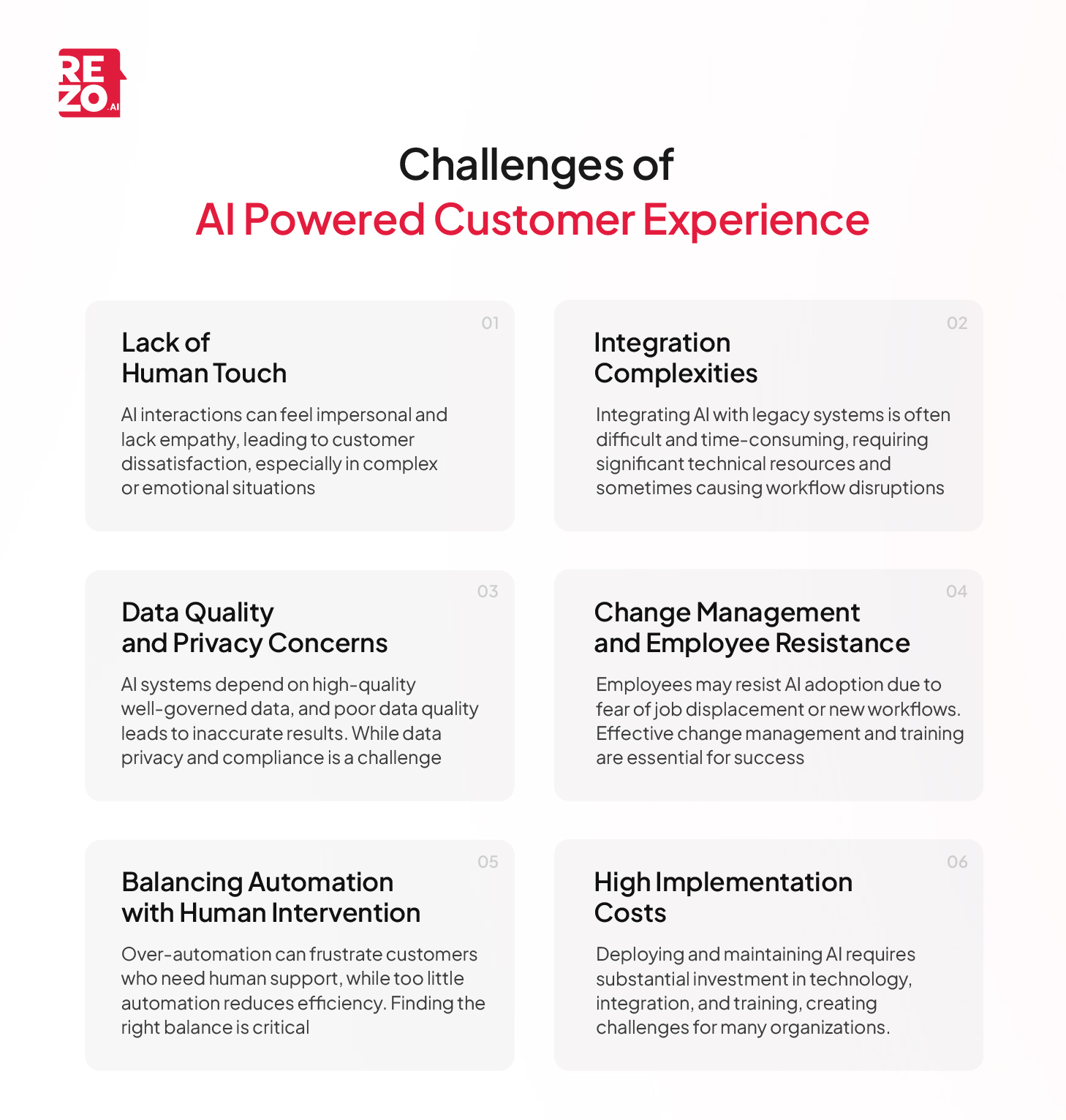
Lack of Human Touch
AI customer experience systems, while efficient, often struggle to replicate the empathy and emotional intelligence that human agents provide. This limitation can impact customer satisfaction, particularly during complex or emotionally charged interactions.
The challenge extends beyond simple communication to include nuanced understanding of customer needs. AI may misinterpret subtle cues or fail to recognize the emotional context behind customer inquiries. This disconnect can lead to frustration and diminished customer loyalty, particularly when customers feel they're being processed rather than understood.
Organizations must carefully design AI implementations that complement rather than replace human interactions. Successful approaches include enabling smooth transitions between AI and human agents, personalizing AI-driven interactions, and ensuring AI conversations feel natural and intuitive.
Integration Complexities
Integrating AI technologies with existing customer service systems presents significant technical challenges. Many organizations operate with legacy systems that weren't designed to accommodate modern AI capabilities, creating compatibility issues that can disrupt customer experience.
Data silos further complicate integration efforts. Customer information often exists in disparate systems across marketing, sales, and service departments. Without unified data, AI systems cannot develop comprehensive customer profiles or deliver consistent experiences across touchpoints.
Organizations can address these challenges through strategic use of APIs and middleware to connect legacy systems with AI technologies. This approach allows companies to implement AI solutions without undertaking complete system overhauls, which can be time-consuming and resource-intensive.
Overcoming Customer Trust Issues
Building customer trust in AI-powered interactions remains a significant challenge. Many customers express skepticism about the reliability and accuracy of AI-driven solutions, particularly when handling sensitive information or complex requests.
Transparency is crucial for establishing trust. Companies must clearly communicate how AI influences customer interactions, what data is being used, and the benefits it provides.
According to a Deloitte research, 73% of consumers are willing to share more personal information if companies are transparent about how it's used. (Source)
Organizations should consider allowing customers to opt in or out of AI-driven interactions, respecting their preferences while gradually building confidence in automated systems. This approach acknowledges customer concerns while demonstrating the value AI can provide.
Balancing Automation with Human Intervention
Finding the optimal balance between AI automation and human involvement presents a significant challenge. Excessive automation can lead to impersonal experiences and customer frustration, while insufficient automation fails to deliver efficiency benefits.
The key lies in strategic deployment of AI for appropriate use cases. Routine, transactional interactions often benefit from automation, while complex, emotionally charged situations typically require human intervention. Organizations must develop clear guidelines for when and how AI should escalate issues to human agents.
Successful implementation involves viewing AI as a force multiplier for human agents rather than a replacement. This perspective focuses on using AI to handle repetitive tasks while enabling human agents to deliver higher-value service that requires empathy and critical thinking.
Data Quality and Management
AI systems are only as effective as the data they're trained on. Poor-quality or biased data leads to inaccurate predictions and potentially discriminatory outcomes that can damage customer relationships and brand reputation.
Organizations must invest in robust data governance practices to ensure AI systems have access to high-quality, diverse, and unbiased datasets. This includes regular data auditing, cleansing processes, and ongoing monitoring for potential biases.
Cost and Resource Constraints
Implementing AI for customer experience requires significant investment in technology, talent, and organizational change. These costs can be prohibitive, particularly for smaller organizations with limited resources.
The financial burden includes not only initial implementation but also ongoing maintenance, training, and system updates.
Organizations can address this challenge by conducting thorough cost-benefit analyses, exploring scalable solutions like cloud-based services, and prioritizing AI projects based on their potential impact on customer experience and business outcomes.
Resistance to Change
Organizational resistance presents a significant barrier to successful AI implementation. Employees may fear job displacement or struggle to adapt to new workflows and technologies.
Addressing this challenge requires comprehensive change management strategies. Organizations should provide adequate training to all stakeholders, involve employees in the implementation process, and address concerns transparently.
By demonstrating how AI enhances rather than replaces human roles, organizations can build internal support for implementation initiatives and ensure smoother adoption across customer-facing teams.
Understanding these challenges is the first step toward developing effective implementation strategies. Organizations that proactively address these obstacles can maximize the benefits of AI while minimizing disruption to customer experience.
Implementing AI in Your CX Process
Successful AI implementation in customer experience requires a structured approach focused on business objectives, integration capabilities, and continuous optimization. The following framework provides actionable steps to incorporate AI into your CX strategy.
Set Clear Objectives
Begin by identifying specific customer experience goals that AI can help achieve. Whether improving response times, personalizing interactions, or automating repetitive tasks. Defining clear objectives will guide your technology selection and implementation strategy. These objectives should align with broader business goals and address existing pain points in your customer journey.
Establish measurable key performance indicators (KPIs) to track progress and demonstrate ROI. Common metrics include customer satisfaction scores, response times, resolution rates, and operational cost reduction. These metrics provide a baseline for evaluating AI effectiveness and justifying continued investment.
Select Appropriate AI Technologies
Choose AI solutions that directly address your identified objectives and integrate with existing systems. Consider these primary technology categories:
- Conversational AI: Chatbots and virtual assistants for handling routine inquiries
- Predictive Analytics: Tools that forecast customer needs and behaviors
- Speech Analytics: Customer interaction analytics on voice of customer, agent performance, call analytics
- Natural Language Processing: Systems that analyze customer sentiment and intent
- Robotic Process Automation: Solutions that streamline back-office operations
Evaluate each technology based on scalability, integration capabilities, and alignment with your specific use cases. Prioritize solutions that offer flexibility and can adapt to changing business requirements.
Map the Customer Journey
Conduct a comprehensive mapping of customer touchpoints to identify optimal areas for AI integration. This process reveals opportunities where AI can deliver the most significant impact, from personalized recommendations to real-time support interventions.
Focus on high-volume, routine interactions that can benefit from automation while identifying complex scenarios that should remain with human agents. This balance ensures efficient resource allocation while maintaining service quality for situations requiring empathy and critical thinking.
Prepare Your Data Infrastructure
AI systems require high-quality, accessible data to function effectively. Evaluate your current data infrastructure and address any limitations before implementation:
- Consolidate customer data from disparate systems
- Establish data governance protocols to ensure quality and compliance
- Implement security measures to protect sensitive information
- Create processes for ongoing data maintenance and updates
Organizations with mature data practices report significantly higher success rates with AI implementation compared to those with fragmented or low-quality data resources.
Train and Fine-tune AI Models
Develop AI models using relevant customer interaction data from chat logs, email exchanges, and call transcripts. This training process involves:
- Data collection and categorization
- Model parameter adjustment for accuracy
- Continuous refinement based on new interactions
- Testing for response accuracy, relevance, and tone
Engineers typically lead this technical process, but CX leaders should remain involved to ensure the AI reflects brand voice and customer service standards.
Integrate with Existing CX Channels
Seamlessly connect AI solutions with your current systems, including CRM platforms, live chat interfaces, and email systems. This integration ensures consistent customer experiences across channels and prevents information silos that could undermine AI effectiveness.
Technical teams should focus on API integrations, data synchronization, and thorough testing to prevent errors or disruptions to customer service operations during implementation.
Ensure Regulatory Compliance
Maintain strict adherence to relevant data protection regulations, including:
- General Data Protection Regulation (GDPR)
- California Consumer Privacy Act (CCPA)
- Industry-specific requirements (HIPAA, PCI DSS)
Regularly review and update privacy policies as regulations evolve, and implement transparent data usage practices that build customer trust. This proactive approach prevents compliance issues that could disrupt AI operations or damage brand reputation.
Train Your Team
Prepare customer service staff to work effectively alongside AI systems. Training should cover:
- Technical operation of AI tools
- Understanding AI capabilities and limitations
- Protocols for handling escalated interactions
- Contributing to AI improvement through feedback
Organizations that invest in comprehensive training report higher employee adoption rates and more effective AI utilization compared to those that neglect this critical step.
Measure Performance and Optimize
Establish a continuous improvement cycle based on performance metrics and feedback:
- Monitor KPIs against established benchmarks
- Collect feedback from customers and employees
- Identify patterns in AI failures or escalations
- Implement regular model updates and refinements
This ongoing optimization ensures AI systems evolve with changing customer expectations and business requirements, maximizing long-term value.
Start Small and Scale Strategically
Begin with focused pilot projects that address specific use cases before expanding to broader implementation. This approach allows for testing and refinement with minimal disruption while building organizational confidence in AI capabilities.
As pilot projects demonstrate success, gradually expand AI implementation across additional customer touchpoints and use cases, applying lessons learned from initial deployments to improve subsequent iterations.
By following this structured implementation framework, organizations can effectively integrate AI into their customer experience processes, realizing benefits while minimizing common implementation challenges.
Conclusion
AI has fundamentally reshaped the customer experience landscape, offering organizations the ability to deliver more efficient, personalized, and proactive service across every touchpoint. The integration of AI enables round-the-clock support, automates repetitive tasks, and provides actionable insights that drive better decision-making and operational efficiency. With AI, businesses can anticipate customer needs, tailor interactions at scale, and ensure consistent service quality, resulting in higher satisfaction and loyalty.
However, realizing these benefits requires careful navigation of challenges such as maintaining the human touch, managing integration complexities, ensuring data quality, and building customer trust. Success hinges on a balanced approach, leveraging AI for automation and insights while preserving opportunities for meaningful human engagement.
For CX leaders and C-level executives, the path forward is clear: prioritize strategic AI implementation, invest in robust data infrastructure, and foster a culture that embraces both technological innovation and customer-centric values. By doing so, organizations can unlock the full potential of AI in customer experience, achieving sustainable competitive advantage in an increasingly digital marketplace.
Frequently Asked Questions
What is the future of AI in customer experience?
Artificial intelligence is projected to handle most customer interactions, enabling hyper-personalized, 24/7 support and more empathetic, human-like experiences. Companies using AI will see improved efficiency, loyalty, and customer satisfaction
How can AI be used in customer success?
AI automates routine tasks, analyzes customer data to predict needs or churn, and delivers proactive, personalized support. This helps teams focus on complex issues and boosts overall customer satisfaction.
What is an example of use of AI for improving customer experience?
Livguard utilised Rezo's AI virtual assistants to automate 66% of the inbound customer interactions. This helped Livguard optimize its contact center efficiency by 3.46x, increase their customer satisfaction by 22.4% and reduce TCO by 37.6%.
Frequently Asked Questions (FAQs)
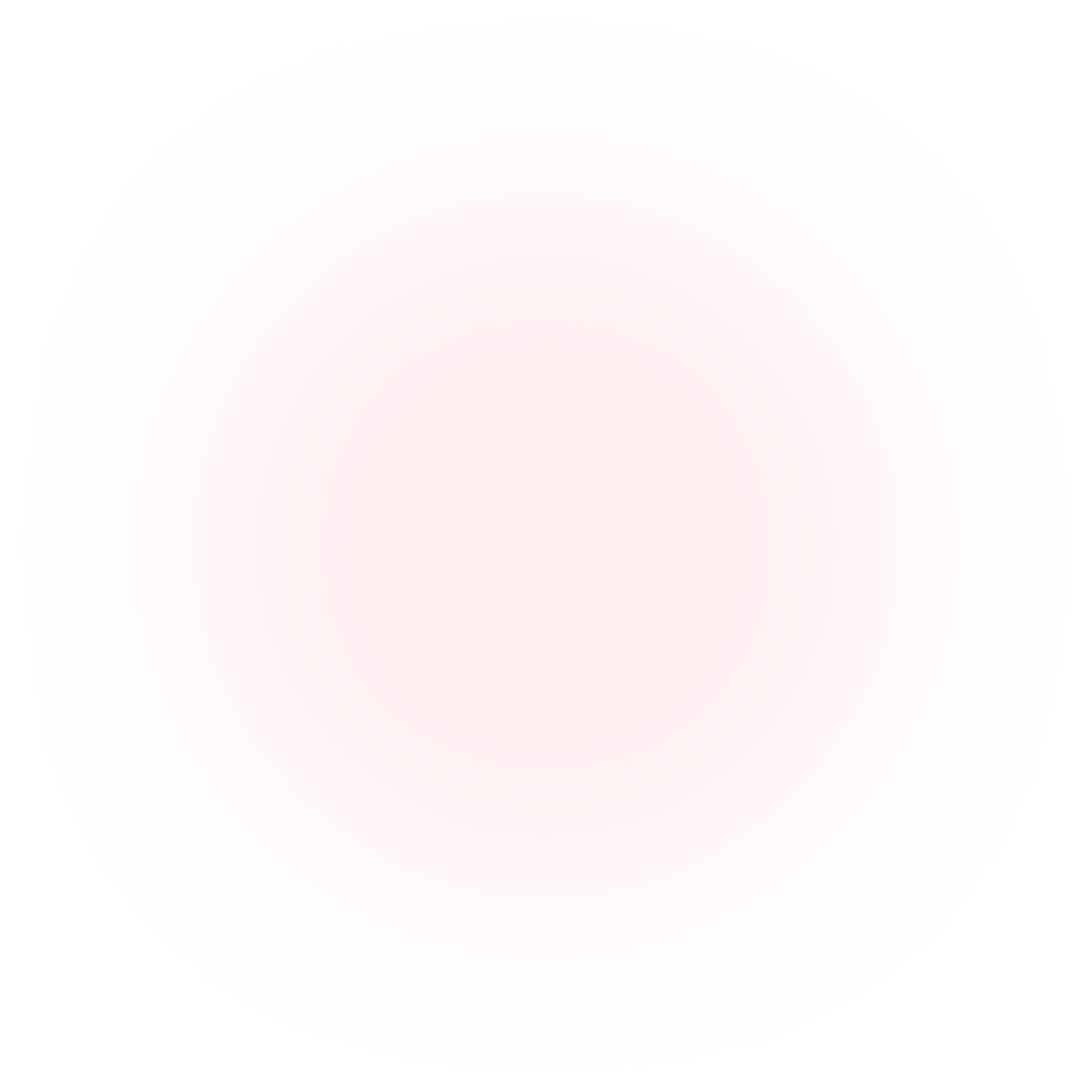